Blogs. Webinars. LinkedIn. Everywhere you turn, you’ve been encountering AI for sales (and with good reason). The list of why you should use sales AI is long and ever-growing. Whether for prioritizing your leads, automating mundane tasks, or forecasting and analytics, AI for sales is here to stay for the long haul.
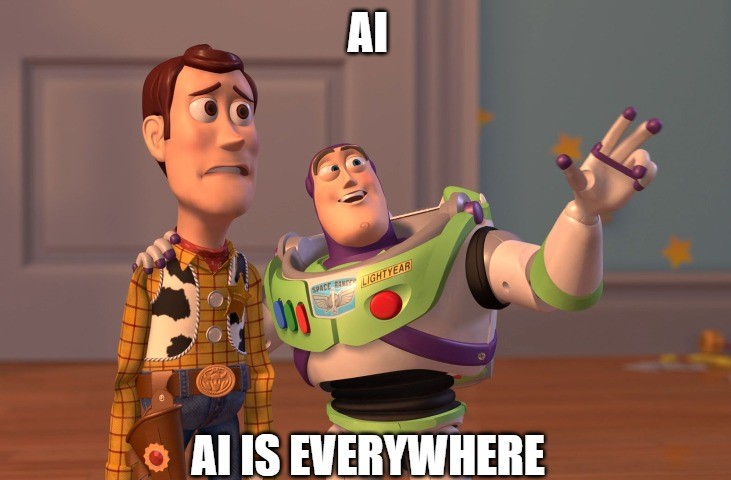
But there’s more. 80% of companies that used AI in sales and marketing reported an increase in revenue, according to McKinsey’s State of AI in 2020 report. Nearly 36% of companies also attributed a decline in operating costs to AI projects.
But I hear you asking, “How does this really benefit my team?”
We’ll answer that and then discuss what you need to have in place within your organization to ensure that your Sales AI project is watertight.
In this post, we’ll talk about:
- How can AI help salespeople sell better?
- How to get started with AI for Sales
- AI maturity — Is your org ready for AI?
- Raw material for AI — Data
- Setting up the goalposts — Outcomes, metrics
- Data science roadmap
- Adoption — Bracing for change
- Key takeaways
How Can AI Help Salespeople Sell Better?
Numerous surveys show that AI is boosting topline revenues and improving conversion rates, but what does it really do for your team?
A Gartner report on the Future of Sales in 2025, brought to you by Freshworks, says that by 2025, AI-guided selling will have four components:
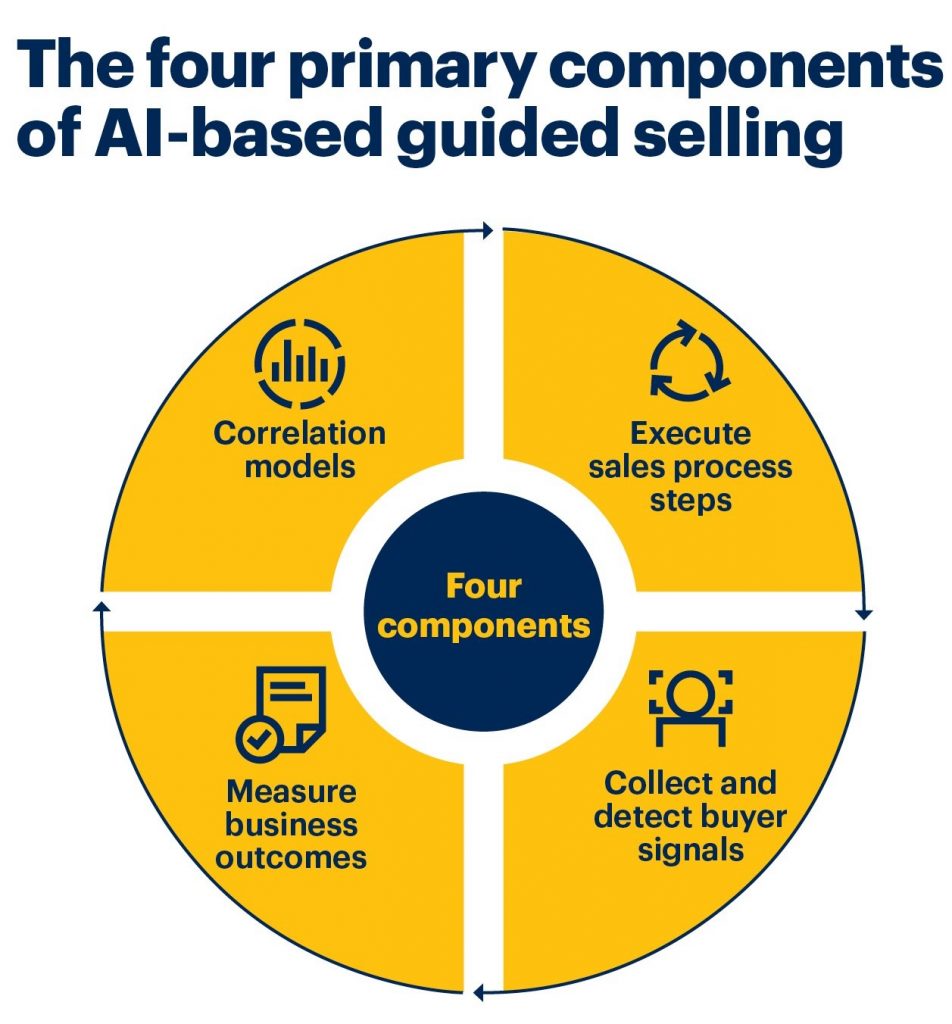
Source
But these are on a higher level and indicate strategic elements. Let’s get down to the more practical aspects. There are two recent Harvard Business Review reports that really map the use-case terrain for AI in sales.
The first one, titled “Accelerating Sales And Marketing Efforts Through Artificial Intelligence”, finds that half the respondents (53%) said that AI allowed their sales teams to “spend less time on administrative activities”.
Data-driven Coaching
About 43% of respondents said that AI helped managers improve their seller productivity through data-driven coaching — they do this by setting goals and benchmarking sales activities based on the team’s top performers. By using data to coach sales reps on activities with the greatest likelihood of a payoff, over 3/4th of salespeople achieved or exceeded their goals.
What Is The Next-best Action?
Another significant benefit of AI noted by 36% of large companies was determining whether sales reps talked to the right people. AI can tell you, based on previous interactions, which profiles are likely to convert and what you need to do to get to a better position this quarter.
If you are coming to the end of the quarter, people have a lot of crazy ideas for how they can close business, like sending the CEO two dozen cupcakes or parking themselves in the lobby. Now we have the data to tell us the next thing you can do that will substantially improve your chances of success, like get on the calendars of two people in the next ten days. And if you can’t, we can stop kidding ourselves and move on to something else.
– Ryan Toben, SVP of Sales, Gainsight
Target The Right Prospects And Customers
The second HBR survey, “Artificial Intelligence And The Future Of Sales And Marketing”, finds that about half the companies surveyed (49%) used AI to identify and target prospects and customers. An almost equal number (48%) of companies said AI helped them garner insights about prospects and customers.
How does AI do this? Data like previous deals and customer profiles can help salespeople identify and prioritize deals that are most likely to convert. Given the customer profile, this will turn the sales folks’ attention to the ‘right’ deals, which have a high probability of conversion.
Tools like AI-powered sales assistants can give salespeople the insights to determine how the deal is faring and the next-best-action on a particular deal based on historical data.

How to get started with Sales AI
Before you jump onto the AI bandwagon, you need to understand that AI is not just a plug-and-play solution. It will not magically fetch you what you’re looking for. Instead, it’s a process that needs to be tailored to your organization and your problem, and it needs to be tuned into your data.
Here are the five major things you need before you delve into an AI project.
1. Training Data, The Raw Material For AI
Data is the fuel that runs your AI engine. And it’s not just the quantity of data. You need to have error-free, diverse, and reliable data sources. Accuracy of the data matters because the AI algorithms take into account past data. The AI needs to be trained and tuned using your organization’s data to get the most accurate recommendations for your particular industry, size, and nature of business.
Let’s take an example to understand the data required. AI can predict your sales for the next four weeks. But only if you have many months of granular, historical data. If all you have is the last few weeks’ data, AI will not help. Instead, you can use a simple forecasting technique like extrapolation.
To identify where exactly your organization stands on data maturity, Dell has a valuable model that you can refer to.
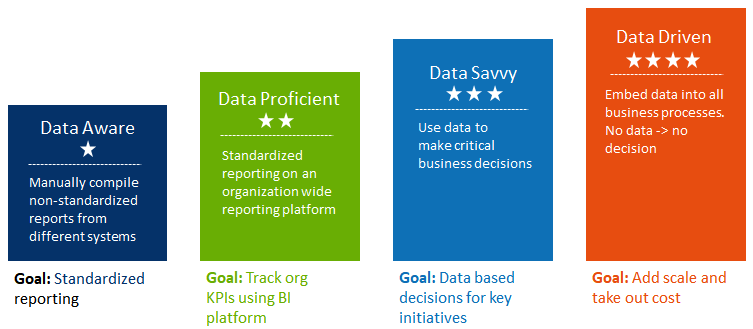
The Dell Data Maturity Model
Most organizations are still at the data-aware or the data proficient stage in the above model. Gartner research shows that 87% of organizations have low levels of BI & Analytics maturity. This means that most organizations still perform spreadsheet-based analyses or pursue data and analytics initiatives as stand-alone projects and in silos.
Ideally, the data requirements for an AI initiative are such that your organization will need to be at least at the data-proficient or data-savvy level in the Dell data maturity model. And once you start using your sales AI, the more you use it, the more accurate it becomes.
But Where Can I Get The Sales Data?
An advantage of using sales technology is that AI is often bundled into tools like an all-in-one CRM. This means that the AI feeds off the CRM data, and if the data quality of the CRM is good, it means that your predictions are on point.
HBR data shows that one out of two companies found CRM data most beneficial for their sales AI efforts.
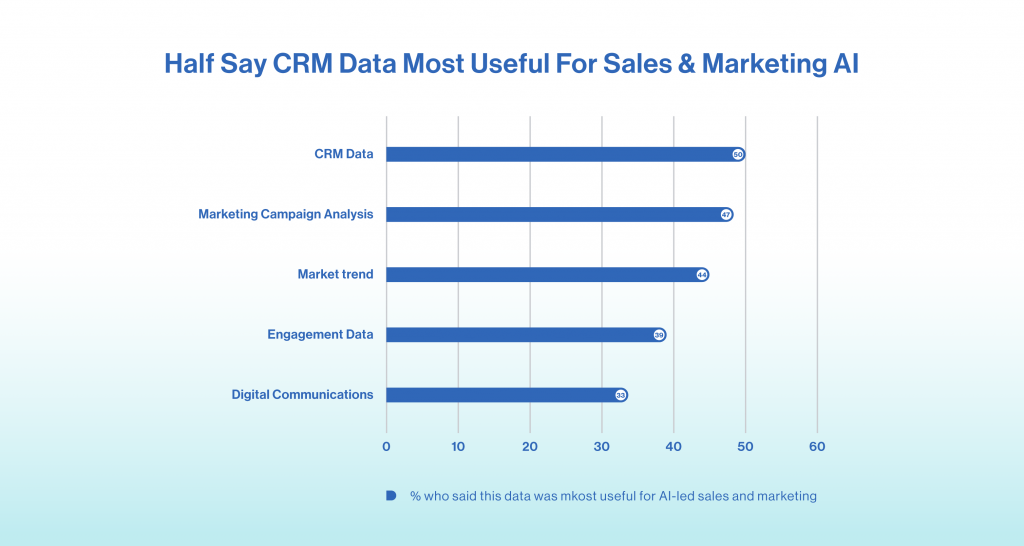
An almost equal number of companies said that marketing campaigns and market trends were two other good data sources.
Data from marketing campaigns can also be tracked by a unified CRM, becoming raw material for AI to answer questions like, “Which type of customers respond well to campaigns and also end up closing deals?”. Answers to these questions help both sales and marketing fine-tune their modus operandi.
2. Identifying The Right Business Use Case
To start with, it’s best to choose a use case that will be a small and easy win. You can then use this to get stakeholder buy-in for future projects and set the stage to inculcate the data culture required for more complicated projects with more significant outcomes.
Let’s look at a few use cases where AI is used. According to the HBR survey, companies primarily use AI in sales and marketing to identify the right customers/prospects and get insights into them.
From a sales perspective, 36% of companies use AI for forecasting. About 1 out of 3 companies use AI for lead scoring and prioritization, and automating basic tasks.
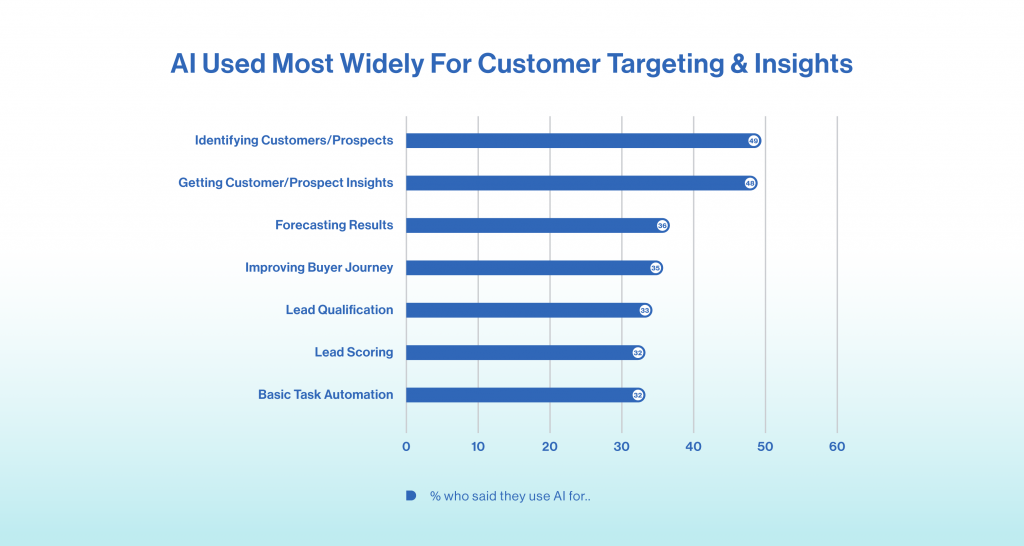
Sample Use Case: AI for Prioritizing Leads
For lead prioritization, there’s no one-size-fits-all approach, as each business has its own priorities.
But you can set up two kinds of scoring priorities: demographic (company size, location, industry) and behavioral (website visits, downloads, email open rates).
For each characteristic or activity, you can add points that work in favor of that lead. For instance, a company size of 500-1000 may be your hot target. If a lead falls into this bucket, you can assign more points.
The sales AI or your AI-powered CRM can help track the lead scores and assign them to reps in real-time.
A potential outcome of this exercise is an increase in closure rates because you went after the best leads. This is a relatively easy use case to go after, one that brings good business value to the table.
Gartner provides a prism that can help you pick out the best use cases based on business value and feasibility.
In the prism, the top use cases have both high business value and are very feasible (lead scoring, for instance).
Other highly feasible sales AI use cases with good business value include:
- Price optimization
- Cross-selling and upselling
- Sales content personalization
3. Setting Up The Goalposts
What does success look like? Is it more leads, more conversions, more sales, and eventually more revenue? To start off with, don’t pick too many metrics. What is the most important metric that you want the AI to affect?
The best way to measure the outcome of a sales AI project is to tie the results to something that you already measure. This way, you will have historical data and benchmarks to compare with your AI-driven outcomes.
You can look at three broad categories while defining what success is:
- Finance Revenue and Pipeline goals: For instance, you can track closure rates (if you’re using AI for lead scoring) and quarterly sales forecasts (if you’re using AI for forecasting). Tracking revenue growth is the most obvious one. Still, it’s sometimes difficult to attribute it to AI because a lot depends on external factors such as how the economy is performing, what other strategic initiatives are being carried out, etc. So choose a metric that you can easily attribute to your sales AI.
- Customer experience: For sales reps, AI can provide a “next-best-action” based on successful deals in the past. For example, nudging a lead with a follow-up to ensure that the lead doesn’t go cold. Based on these actions, you can measure how closure rates changed before and after the introduction of AI. You can even move beyond the traditional customer experience scores or NPS scores and into the realm of Natural Language Processing. You can listen to what your customers are saying in the comment box about their buying experience.
- People goals: Happier sales teams significantly outperform their less happy peers across every conceivable metric, be it hitting quota, revenue growth, or customer satisfaction. According to a Freshworks-Harvard Business Review study, 81% of very happy sales teams reported increases in annual sales over the past two years. Click here to read more on the case for sales happiness as a metric.
You can also go with productivity metrics like “How long does it take for a group of people to achieve a certain outcome before and after AI implementation?” Or “How many hours of non-selling time have been converted to selling time because of automating mundane tasks with AI?
4. Data Science Roadmap
Once you’ve set up the relevant metrics, it’s time to create an actionable roadmap for your sales AI project. Data science company Gramener’s whitepaper on how to pick the best data science project has some answers.
There are two approaches that you should map out — a business-driven and a data-driven approach. Make lists by answering the following:
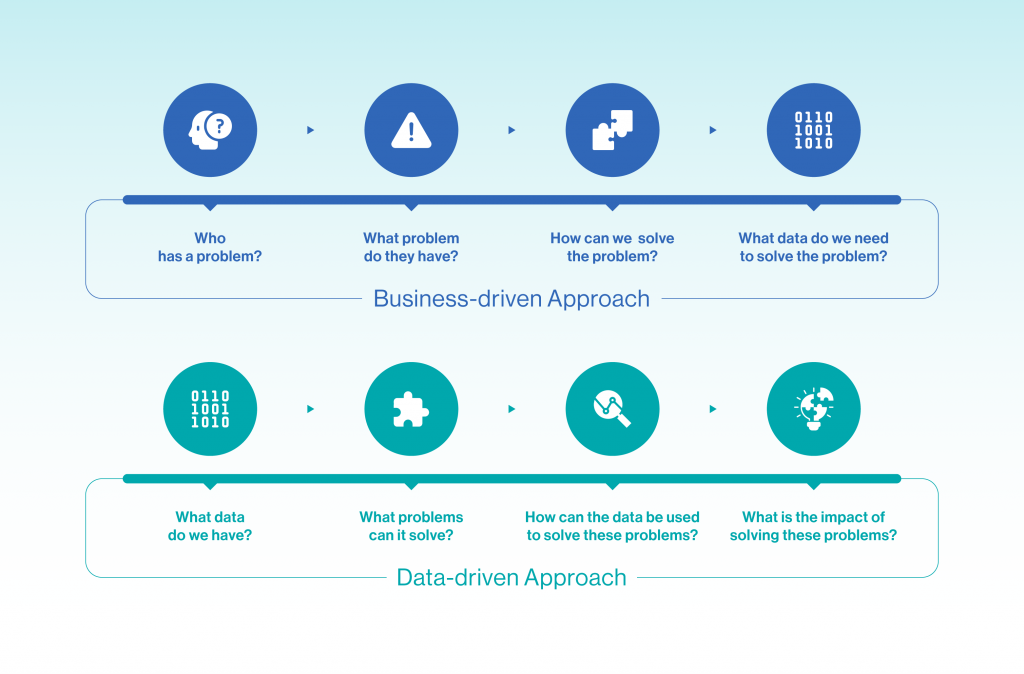
When you map these two approaches, you will find that you may not have the data to solve some of your business problems. You may also have data that does little to solve the problems that you do have.
However, you will also have a sweet spot: Data to solve a list of problems that you do have. Pick these up, and get started with them.
Example Use Case: AI in forecasting
Let’s take the example of using AI in forecasting and run through both approaches. For each question, prepare a list of possible answers and work through them.
In the business-driven approach, we can list the problem of sales leaders having a difficult time predicting revenue. They can get better at this by using AI-led forecasting using data on past deals.
Now, we work through the data-driven flow: Suppose we have data on previous deals. This can help sales leaders predict deals that are likely to close, using techniques like stage duration analysis (how long does it take for a deal of this magnitude to progress through each stage?).
This in turn improves the forecast accuracy of sales leaders. It helps organizations keep a tab on the projected revenue and initiate steps to course-correct when the projected revenues and targets don’t match.
Once you have a data science roadmap in place, it becomes easier to understand what you can and cannot do using sales AI, and what to keep an eye out for.
5. Adoption: Bracing for change
Sales AI is often just a black box for most salespeople, and there’s no solid reason for them to “do as it recommends”. You have to make people understand clearly why it helps them and why they should care.
It often helps to start your AI implementation journey bottom-up. Find out what are the real problems your salespeople are facing that AI can solve.
Be wary of the technology you bring in. The Freshworks-HBR survey on sales happiness found that 71% of respondents agree that a poor software/technology user experience can negatively impact salesperson happiness.
“Too often, sales technology is chosen for sales teams and not with them. The technology chosen does not actually enable salespeople to do their jobs better. Instead, it helps managers track sales activities,” says David Mattson, CEO and President of Sandler Training.
Answer questions for your team before you take up a sales AI project. Basically, have an answer for the question about the specific outcome the AI project helped your team achieve, something that they couldn’t do before. Once that’s done, you can press on with things like setting up roadshows.
Here are five things you can do to ensure adoption.
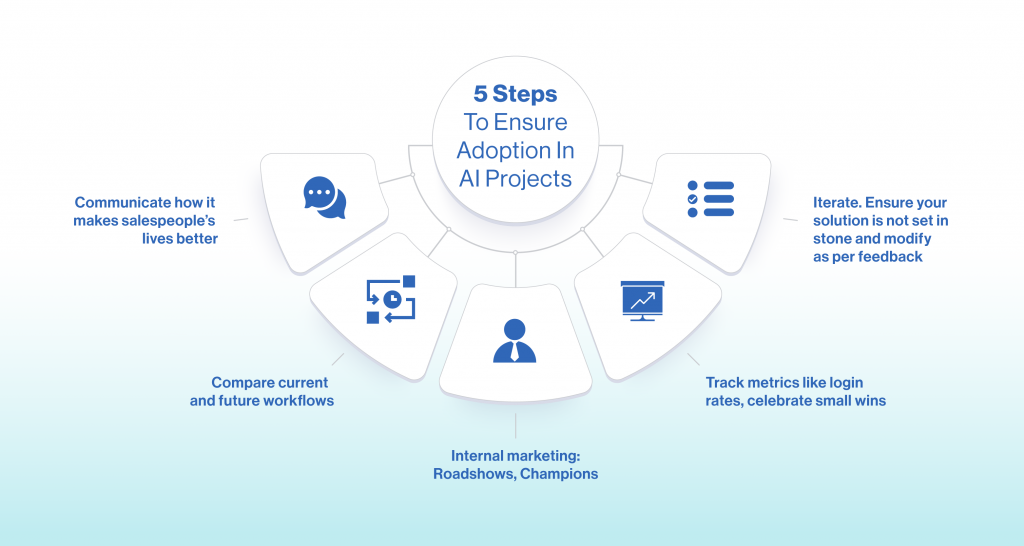
How does it make their lives better? Questions like, “Why are we taking up a sales AI project?” need to be answered on the lines of, “We are moving from Point A to Point B because of C. These are the benefits for your team, and consequently, for our organization.” Try to be as specific as you can. For example, “This lead scoring engine will help you pursue leads who are most likely to convert based on people we have converted in the past. This will put your time to best use and increase your closure rate.”
Where does it fit in? Compare the workflow before AI to the post-implementation scenario. What tasks can reps automate? What new tasks do they have to do, and what can they slash out from their to-do list?
Internal marketing about the project can help you with adoption. Get the word out on the whys and the hows. For example, you can organize a roadshow with champions in each team. Since they are allocated at the team level, there will be less reluctance to approach them with queries.
Track metrics. Adoption metrics like login rates help you understand whether people are actually using the solution, and usage is the most significant indicator of adoption. In addition, you can also track the metrics you set up in step 3 above and celebrate small victories with your team to ensure they stay motivated to use the solution.
Iterate. Ensure your solution is not set in stone. Iteratively modify your solution based on the feedback you receive from your team. This will ensure that the irritants that prevent adoption are weeded out.
Takeaways
We’ve just gone through the five major things you need to have in place before embarking on your sales AI journey — data, use case, metrics, roadmap, and ensuring adoption once the project is live.
One of the most critical success factors lies in the use case you pick for execution. You could choose a use case that would eliminate manual processes (like sending emails) or one that is fraught with human error (like forecasting).
You also need to ensure that your goals are realistic. According to the HBR survey, fewer than half (47%) of those who use AI in sales and marketing have put in place measurable improvement goals to help them assess the impact of their investments — a sub-optimal outcome.
While embarking on an AI project, it’s possible to be “too ambitious—creating ‘moonshot’ projects that never reach the moon,” says Tom Davenport, President’s Distinguished Professor of Information Technology and Management at Babson College.
“It’s better to be in the middle, thinking big, yet starting small with a number of doable projects,” he says.
According to Gartner research, 30% of all B2B companies employ some kind of AI to augment at least one of their primary sales processes. Will you be among them?

Cover image and infographics by Prasanna Bhupendran